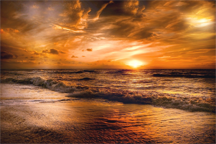
The probability that the exact moment of sunset will be obstructed by clouds is independent of the result of the roll of a die. We can reason about these two events in ways that we cannot reason about events that are not independent.
If making decisions is part of your job, you know that some decisions are far from straightforward. Sometimes we make decisions based on partial information or guesses. For example, when we must choose between A and B, we make estimates of the probabilities of A's success and B's success. Then we pick the one we think best. Sometimes we do this without realizing that this is the process we're using. That's where it gets interesting, because most of us, as humans, are really bad at making decisions that involve probabilities.
This post People make a common mistake
when forming estimates of
the probability of multiple
events occurringprovides an example of a simple decision problem involving probabilities. It's a problem that causes many of us to take a wrong turn. I begin by posing the problem in semi-realistic terms fairly close to something that actually could come up at work. After posing the problem, I take a short excursion into a little refresher of some basic probability methods. Then I'll return to the semi-realistic problem to apply those methods. Should be fun.
A semi-realistic problem
Suppose we're making a risk plan. The risk we're planning for is the risk that members of our team will be stricken with COVID during the next month. For this scenario, an important fact is that our team members are all classified as essential, and we all work in the same suite of offices, using the same break room. That's why we're concerned about COVID.
Our plan is to find a way to meet our deadlines as best we can, even if some of our team fall ill. So we do a bit of cross training and we prepare for several scenarios. Here's our plan:
- If A falls ill, B and C will take over A's responsibilities
- If B falls ill, C and A will take over B's responsibilities
- If C falls ill, A and B will take over C's responsibilities
We estimate that the probability of anybody falling ill during the next month is 10%. So the question is: How well will our plan work?
One thing is clear: if all of A, B, and C fall ill, our plan won't work at all. So our task is to work out the probability that all three of A, B, and C will fall ill.
A little refresher about probabilities
Our semi-realistic problem has three events: A falls ill, B falls ill, and C falls ill. That's a little complicated, so let's take a look at a two-event problem. One event is this:
Event X: When the Sun sets tonight, at the moment of sunset the sun will be obscured by clouds.
The probability: 1/5
And the second event is this:
Event Y: Right after sunset, I roll a die, and it lands so that either one or two spots are showing.
The probability: 1/3
So the question is this: what is the probability that both Events X and Y occur? Call that XY.
Solution: P(X) is 1/5. P(Y) is 1/3. So P(XY) = P(X) times P(Y) = 1/15.
Explanation: X occurs one-fifth of the time. For the other times, it doesn't matter what I get when I roll the die. Only when X occurs does the die matter. So right from the beginning, we're down to P(X)=1/5 of the time as an upper limit for P(XY). But the probability that Y occurs is only 1/3. So P(XY) = 1/3 of 1/5 of the time. That is P(XY) = P(X)*P(Y) = 1/15.
So here's what we learn from this: If Event X and Event Y are independent of each other, P(XY) = P(X) * P(Y). This isn't a proof. It's a plausibility argument. I'll leave the proof to the mathematicians.
It's also plausible to suggest that the number of independent events doesn't matter. If I add a third event Z:
Event Z: I flip a coin and it lands heads side up
The probability: 1/2
Then the probability of XYZ = P(XYZ) = P(X)*P(Y)*P(Z) = 1/15 * 1/2 = 1/30.
The generalization of what we've learned is this:
The probability that every one of a set of independent events occurs is the product of the probabilities of the members of the set of events.
Now we're ready to return to our semi-realistic problem.
Solving our semi-realistic problem
To compute the probability that our risk plan will fail totally, many would argue as follows. If P(A), P(B), and P(C) are respectively the probabilities for A, B, and C falling ill, then the probability of all three falling ill would be P(A,B,C) = P(A)*P(B)*P(C) = 0.1*0.1*0.1 = 0.001. So by this calculation, our plan will be workable 999 times out of 1000.
Unfortunately, in the real world, that would be wrong. By a lot.
What's wrong with our reasoning is this. To find the probabilities of all of A, B, and C falling ill, we multiplied their respective probabilities together. The theorem we're applying (actually misapplying) is, as we saw above:
The probability that every one of a set of independent events occurs is the product of the probabilities of the members of the set.
But in this case, the probabilities of the three events aren't independent.
That is, the probability that B falls ill, given that A is ill, is no longer just 10%, because all three work in the same office. One can infect the others. We might not know what the probability actually is, but it's more than 10%. The same is true for C. The probability that C falls ill, given that A is ill, is greater than 10%. Computing the actual result is difficult, but we can tell right away that the probability of all three falling ill is much larger than 1/1000.
Last words
If you surf the Web much, you'll find numerous examples of misapplication of our probability rule for independent events. The rule works well for independent events. But to apply it to multiple events needs justification. Verify that the events are independent before using the method. Top
Next Issue
Projects never go quite as planned. We expect that, but we don't expect disaster. How can we get better at spotting disaster when there's still time to prevent it? How to Spot a Troubled Project Before the Trouble Starts is filled with tips for executives, senior managers, managers of project managers, and sponsors of projects in project-oriented organizations. It helps readers learn the subtle cues that indicate that a project is at risk for wreckage in time to do something about it. It's an ebook, but it's about 15% larger than "Who Moved My Cheese?" Just . Order Now! .
Your comments are welcome
Would you like to see your comments posted here? rbrenjTnUayrCbSnnEcYfner@ChacdcYpBKAaMJgMalFXoCanyon.comSend me your comments by email, or by Web form.About Point Lookout
Thank you for reading this article. I hope you enjoyed it and
found it useful, and that you'll consider recommending it to a friend.
This article in its entirety was written by a human being. No machine intelligence was involved in any way.
Point Lookout is a free weekly email newsletter. Browse the archive of past issues. Subscribe for free.
Support Point Lookout by joining the Friends of Point Lookout, as an individual or as an organization.
Do you face a complex interpersonal situation? Send it in, anonymously if you like, and I'll give you my two cents.
Related articles
More articles on Effective Communication at Work:
Practice Positive Politics
- Politics is a dirty word at work, as elsewhere. We think of it as purely destructive, often distorting
decisions and leading the organization in wrong directions. And sometimes, it does. Politics can be
constructive, though, and you can help to make it so.
What, Why, and How
- When solving problems, groups frequently get stuck in circular debate. Positions harden even before
the issue is clear. Here's a framework for exploration that can sharpen thinking and focus the group.
That Was a Yes-or-No Question: I
- In tense situations, one person might question another. As the respondent replies, the questioner interjects,
"That was a yes-or-no question." The intent is to trap the respondent. How does this work,
and how can the respondent escape the trap?
Anticipate Counter-Communication
- Effective communication enables two parties to collaborate. Counter-communication is information provided
by a third party that contradicts the basis of agreements or undermines that collaboration.
Interrupting Others in Meetings Safely: I
- In meetings we sometimes feel the need to interrupt others to offer a view or information, or to suggest
adjusting the process. But such interruptions carry risk of offense. How can we interrupt others safely?
See also Effective Communication at Work and Effective Communication at Work for more related articles.
Forthcoming issues of Point Lookout
Coming February 26: Devious Political Tactics: Bad Decisions
- When workplace politics influences the exchanges that lead to important organizational decisions, we sometimes make decisions for reasons other than the best interests of the organization. Recognizing these tactics can limit the risk of bad decisions. Available here and by RSS on February 26.
And on March 5: On Begging the Question
- Some of our most expensive wrong decisions have come about because we've tricked ourselves as we debated our options. The tricks sometimes arise from rhetorical fallacies that tangle our thinking. One of the trickiest is called Begging the Question. Available here and by RSS on March 5.
Coaching services
I offer email and telephone coaching at both corporate and individual rates. Contact Rick for details at rbrenjTnUayrCbSnnEcYfner@ChacdcYpBKAaMJgMalFXoCanyon.com or (650) 787-6475, or toll-free in the continental US at (866) 378-5470.
Get the ebook!
Past issues of Point Lookout are available in six ebooks:
- Get 2001-2 in Geese Don't Land on Twigs (PDF, )
- Get 2003-4 in Why Dogs Wag (PDF, )
- Get 2005-6 in Loopy Things We Do (PDF, )
- Get 2007-8 in Things We Believe That Maybe Aren't So True (PDF, )
- Get 2009-10 in The Questions Not Asked (PDF, )
- Get all of the first twelve years (2001-2012) in The Collected Issues of Point Lookout (PDF, )
Are you a writer, editor or publisher on deadline? Are you looking for an article that will get people talking and get compliments flying your way? You can have 500-1000 words in your inbox in one hour. License any article from this Web site. More info
Follow Rick





Recommend this issue to a friend
Send an email message to a friend
rbrenjTnUayrCbSnnEcYfner@ChacdcYpBKAaMJgMalFXoCanyon.comSend a message to Rick
A Tip A Day feed
Point Lookout weekly feed
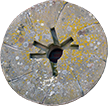